Quantifying RL Policies and Environments
Jun 19, 2021
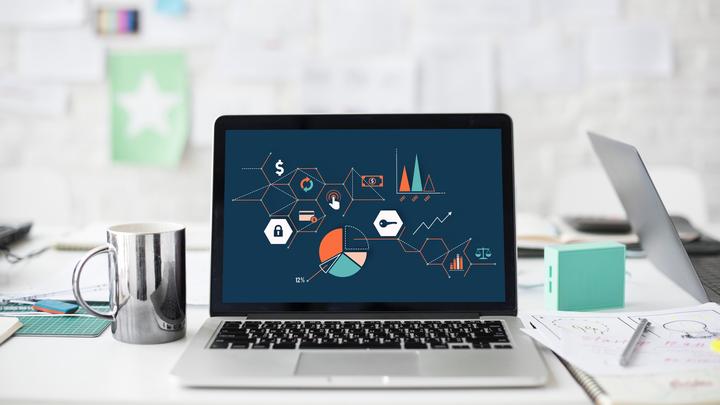
Related
- Continuous-Time Lagrange Relaxation for Systems with Delays
- Non-Ideal Fixed Points in Self-Repairing 3D Printers
- Robot Showcase Project
- (DEMETER) Development of adaptive pruning robots for sustainable viticulture through simulation empowered AI, robot learning and Intuitive TeleopeRation
- AI Application Project (2023 Autumn)