Our Ecology Theory of RL workshop is accepted to NeurIPS 2021!
Our Ecology Theory of RL workshop is accepted to NeurIPS 2021!
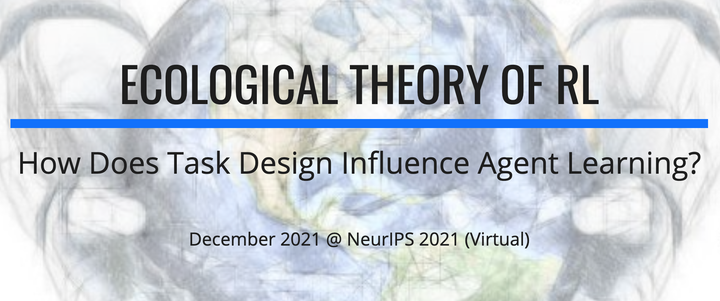
We are co-organizing the first workshop on the Ecological Theory of Reinforcement Learning @ NeurIPS 2021, with researchers from UC Berkeley, CMU, U of Montreal, U of Washington, U of Amsterdam, and Google Brain! We focus on data-centric view on RL, and solicit papers that study how task designs influence agent learning.
CALL FOR PAPER
In reinforcement learning (RL), designing general-purpose algorithms that apply to arbitrary Markov Decision Processes (MDPs) is very appealing because it broadens the range of problems that we can address using this technique. However, when we utilize these methods to solve real applications, we put considerable time into carefully parameterizing the problem, such as selecting the appropriate state representations and action spaces, fine-tuning reward functions, and designing data collection strategies. RL is not alone in this regard: researchers in the supervised learning community typically assume datasets to be fixed (and iterate on the algorithms and models), while practitioners often fix the algorithm and model (and instead iterate on the dataset). Some have argued that perhaps a more data-centric view of machine learning research is needed [18, 12], and we would like to encourage the research community to investigate this same principle in the context of RL.
Data in RL may be understood to be the properties of environments and tasks, usually modelled through underlying MDPs. From this perspective, a data-centric study of RL would parallel Gibson’s ecological theory of visual perception and psychology [9]. An ecological study of RL should examine the behavior of algorithms in the context of their environment to further understand how different properties (such as linearity, ergodicity, mixing rate, among others) influence the performance of these methods. We want the community to develop a systematic approach to RL task design that complements today’s algorithmic-centric view. Properties and taxonomies of environments and tasks have been previously investigated in several areas of RL research such as curriculum and continual learning [27, 5, 22], bisimulations and homomorphisms [21, 7, 4, 26], affordances [28], PAC analysis [13, 1], information-theoretic perspectives [14, 11, 16, 8], meta-analysis of RL benchmarks [17, 20, 19], among many others. However, these endeavors have been usually disconnected from the efforts made to build environments and tasks [2, 25, 3, 24, 23, 6, 15, 10 ], leaving a gap in our understanding of how algorithmic solutions and environments designs interact.
This workshop builds connections between different areas of RL centered around the understanding of algorithms and their context. We are interested in questions such as, but not limited to:
- How to gauge the complexity of an RL problem.
- Which classes of algorithms can tackle which classes of problems.
- How to develop practically applicable guidelines for formulating RL tasks that are tractable to solve.
We expect submissions that address these and other related questions through an ecological and data-centric view, pushing forward the limits of our comprehension of the RL problem. In particular, we encourage submissions that investigate the following topics:
- Properties and taxonomies of MDPs, tasks or environments and their connection to:
- Curriculum, and continual, and multi-task learning
- Novelty search, diversity algorithms, and open-endedness.
- Representation learning.
- MDPs homomorphism, bisimulation, inductive biases, equivalences and affordances.
- PAC analysis of MDPs.
- Dynamical systems and control theory.
- Information-theoretic perspectives on MDPs.
- Reinforcement Learning benchmarks and their meta-analyses.
- Real-world applications of RL (Robotics, Recommendation, etc.)
Properties of agents' experiences and their connection to:
- Offline Reinforcement Learning.
- Exploration.
- Curiosity and intrinsic motivation.
- Skills discovery and hierarchical reinforcement learning.
- Unsupervised objectives for reinforcement learning.
IMPORTANT DATES
- Submissions Open: Aug 1, 2021 00:00 AOE
- Submissions Deadline: Sep 17, 2021 23:59 AOE
- *Authors Notification: Oct 22, 2021
- Camera Ready: Nov 1, 2021