RT-1:マルチモーダルなロボティクス基盤モデルへの期待 ~Robotics Transformer 1~
「基盤モデル×Robotics」 Advent Calendar 2022 15日目
はじめに
「基盤モデル×Robotics」のAdvent Calendarにご参加して頂き, ありがとうございます! 本記事は東京大学松尾豊研究室のサブグループであるTRAILのテックブログでご紹介させて頂いております!
今回の記事では,Robotics at Google, Everyday Robots, Google Research, Brain Teamが提案した 「RT-1: Robotics Transformer for Real-World Control at Scale」 を紹介します. arXivには2022年12月14日(日本時間)に投稿されています。 この記事が2022年12月15日投稿なので、かなりホットな話題ではないでしょうか?
私の専門はもとより制御、メカトロニクス、ロボティクスなのですが、 AI領域(知能)も数年前から興味があり、取り組んでいます。
まだまだ至らない点もあるかと思いますが、2022/12/14-12/15でRT-1を読んで、記事を2022/12/15に投稿なので、 少し温かい目で頂ければと思いますm(- -)m (Happy Birthday to Me…誕生日にRT-1に出会えて幸せでした笑)
(ちなみに、、、2022年12月14日に松嶋達也さんからRT-1の存在を教えて頂いた時は衝撃がとまらず、ワクワクしました!)
「基盤モデル×Robotics」アドベントカレンダーでは、松嶋達也さんの基盤モデルであるCLIP記事、も面白いので是非ご覧ください! (東京大学松尾豊研究室もロボットばりばりやってます!)
少し脱線しましたが、、、 それでは、RT-1が作り出す世界観を是非とも楽しんで頂ければと思います。
*少々私の「ひとりごと」が入っている箇所があるかもしれませんが、 その際は、私個人の意見・思想としてあたたかい目で見て頂けると幸いです。
目次
RT-1とは
RT-1概要
「Robotics Transformer 1」を略してRT-1と名付けられています。
RT-1はRoboticsの基盤モデルになりうるポテンシャルを大いに秘めてるのではないかと思います。(既になっている?)
(勝手に名付けますが、Robotics Foundation Model (RFM)と呼ばれる日が、もしかしたら近いかもしれません。)
下の動画がRT-1のイメージ動画となっております。
私自身、最初にこの動画を見た時、正直…「なんだこれは?!」となりました。(色々な意味で)
本記事を最後までお読み頂ければ、あっ!「スポンジ!」みたい!となるかもしれません。 (スポンジのようにどんどん吸収してRT-1が一般化していきます。要するに単一モデルで様々(約740)なタスクが可能となりました。)
で、RT-1は何ができるの?良いの?となるかと思いますので、 百聞は一見にしかずということで、 ひとまずRT-1の素晴らしいYoutubeを是非見てください。
約9分の動画ですが、これ以降のブログを読む前に見ることをオススメします!(これを見たらブログ読まなくて…も…)
ご覧頂いたように、以下が動画の概要です。
大規模なロボットデータ(17ヶ月、13台のロボット、700task、130k episodes)を用いた学習モデル「RT-1」
RT-1の学習に他のロボット(論文ではKUKA)、Sim2Real,Simデータを活用することでTask成功率が向上
SayCanとRT-1による動作実現
等あります。
↑専門用語多すぎるとなるかもしれませんが、各リンクへ飛んで頂ければと思います。
少し寄り道ですが、 RT-1の論文を読んで、個人的な衝撃(驚き)は以下でした。
長期間、大量のデータ収集 (デモ数:130k、使用ロボット(EDR):13台、Task数:744、収集期間:17ヶ月)や700 skills at a 97% success rate
Actionの内容(arm, baseのposition等を考慮)&Mobile Manipulator (EDR)で実現
RT-1の周期が3[Hz]
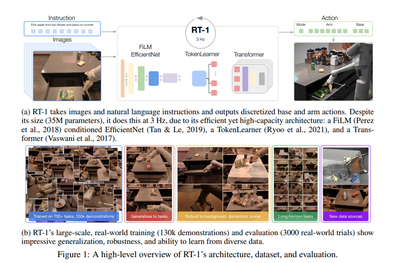
他のロボット(論文ではKUKA)、Sim2Real,Simデータを活用可能することでタスク成功率が向上(ある意味で)
SayCanとRT-1の併用
個人的には数え切れないくらいあるんですが、この辺りにしておきます。 “sponge up”, “generalize"なども評価もしっかりしてます。
RT-1の著者様
さて、RT-1は、、、 Robotics at Google, Everyday Robots, Google Research, Brain Teamによって提案されました。 僭越ながら以下に著者様を記載させて頂きます。
Anthony Brohan, Noah Brown, Justice Carbajal, Yevgen Chebotar, Joseph Dabis, Chelsea Finn, Keerthana Gopalakrishnan, Karol Hausman, Alex Herzog, Jasmine Hsu, Julian Ibarz, Brian Ichter, Alex Irpan, Tomas Jackson, Sally Jesmonth, Nikhil J Joshi, Ryan Julian, Dmitry Kalashnikov, Yuheng Kuang, Isabel Leal, Kuang-Huei Lee, Sergey Levine, Yao Lu, Utsav Malla, Deeksha Manjunath, Igor Mordatch, Ofir Nachum, Carolina Parada, Jodilyn Peralta, Emily Perez, Karl Pertsch, Jornell Quiambao, Kanishka Rao, Michael Ryoo, Grecia Salazar, Pannag Sanketi, Kevin Sayed, Jaspiar Singh, Sumedh Sontakke, Austin Stone, Clayton Tan, Huong Tran, Vincent Vanhoucke, Steve Vega, Quan Vuong, Fei Xia, Ted Xiao, Peng Xu, Sichun Xu, Tianhe Yu, Brianna Zitkovich
上記の皆様には、このような素晴らしい研究成果を世に公開してくださったことに深く感謝いたします。 しかもなんとオープンソースです。コードはこちらにあります。
Very excited to introduce the Robotics transformer 1, a large transformer-based imitation-learned robot manipulation policy, that can execute over 700 skills at a 97% success rate. It can also learn from other robots, execute long horizon SayCan and show massive scaling potential https://t.co/My9hoab0yy
— Keerthana Gopalakrishnan (@keerthanpg) December 13, 2022
EDR (Mobile Manipulator:Everyday Robots) RT-1で使用したロボット
RT-1の論文で使用されている「EDR:Everyday Robots」のモバイルマニピュレータ(ロボット)について少し見てきましょう。 (ロボット好きだと気になってしまいますね!)
特徴
あまり詳細情報を発見できなかったのですが、以下の特徴があるようです。
- Mobile Manipulator(移動と作業ができるロボット)
- 7DoF(7自由度)のロボットアーム搭載
- グリッパ搭載
「Everyday Robots」がこのロボット等について動画を出しているので、 ご興味あれば覗いてみてください。このPart1-4が動画が個人的に良きです。
ロボットが沢山いたり、職場の雰囲気を少し動画で見れるのは良いですね!(youtubeならではって感じです。)
あと、このページも見るとロボットの中とか少し見れるので楽しいですね。
では、RT-1について少し詳しく説明させて頂きます!
背景:何故RT-1が今、この瞬間に誕生したのか
まず何故RT-1のようなモデルがロボティクス領域において、今まで現れてこなかったのでしょうか? 少しこの辺りを探ってみましょう。
ChatGPT
その前に、既にある一部の人は体感されたかと思いますが、 「ChatGPT」をご存知でしょうか?
ChatGPTとはOpenAIが開発するGPT-3という基盤モデルをベースとしたチャットアプリです。まるで人と話しているかのような返答がくるAIです。
ChatGPTに関しては以下の松尾研究室の今井さんの資料がとても詳しいので良ければご覧ください。
またChatGPTはこんなこともできて、個人的にツボでした笑(このツイッター垢は私ではありません)
ChatGPTに「知らんけど」を教えたら早速使ったwww pic.twitter.com/L3nMFIyLSY
— かんちゃんたろう (@kan5r) December 2, 2022
基盤モデル
「ChatGPT」は、テキストを入力すると、まるで人の様な知能があると感じさせられるような返答(テキスト)を生成します。(つっこみが沢山でてきそうな記載ですみません) ChatGPTのようなアプリが実現できた理由の1つとして、アドベントカレンダーでもあつかっている「基盤モデル」の活躍が顕著です。
基盤モデルとは「大量で多様なデータを用いて訓練され, 様々なタスクに適応(ファインチューニングなど)できる大規模モデル」です。2021年にBommasaniらのスタンフォード大学のワーキンググループによって, 基盤モデル foundation modelと命名されました.
こちらの記事「基盤モデルとは」も良ければご覧ください。
「ChatGPT」のようなアプリを作成するには、それのもととなる「GPT-3」が必要になります。 「GPT-3」は175B(1750億)のモデルパラメータを持った基盤モデルとなり、かなり大規模なモデルであることが想像できるかと思います。 それに伴い、学習させるに辺り、大量のデータを必要とします。
幸い(?)ながら言語や画像情報は、言語等の違いはあるものの、 大量のデータはインターネット上にあります。
もうお気づきかもしれませんが、 この「大量のデータ」がロボティクス領域において「肝」になってきます。
ロボットデータ
はい。うすうす感じているかもしれませんが、 ロボットデータがインターネット上に画像や言語のように無数にないのです。 そもそもロボットデータとは何があるのでしょうか?
簡単な例を上げるために、 ここで扱うロボットをモーター駆動のロボットとします。
参考までに以下にロボットデータ例をあげますと、、、
- ロボットの関節角度、速度、トルク
- ロボットの位置、姿勢(手先、脚、身体)
- ロボットハンドの開閉
等などがあります。
興味ある方は、 内界センサ、外界センサ等のワードをググると面白いかもしれません。
異構造ロボット
勘の鋭い方は次のように思うのではないでしょうか? 「あるロボットを用意して永遠にある動作(物を拾う、服をたたむ、等)させておいてデータを集め続けていたら良いのでは?」
これはある意味「脳筋」でロボットデータ集める方法の1つなのですが、 次の図を見てください。
上の図のように、ロボットも沢山種類があります。
- 移動ロボット(車輪、脚等)
- ロボットアーム(単腕、双腕等)
- 移動マニピュレータ(移動ロボットにロボットアームを搭載)
- ヒューマノイドロボット
上記以外にも沢山あります。
つまるところ何が言いたいかと言いますと、 ハードウェア的に異構造のロボット間ではロボットデータを共有することは難しいのです。 なので折角頑張って集めても、ロボットの種類を変えてしまうとデータを共有できないみたいな事がこれまでは多かったです。(一部を除く)
ですが、「RoboNet」,「BC-Z」、「RT-1」等の登場によってその見方も変更しつつあるかもしれません。
是非以下リンクから動画をご覧ください。 なんだか色々と思考が広がり楽しくなってきますね!
Robot learning has emerged as a promising tool for taming the complexity and diversity of the real world. Methods based on high-capacity models, such as deep networks, hold the promise of providing effective generalization to a wide range of open-world environments. However, these same methods typically require large amounts of diverse training data to generalize effectively. In contrast, most robotic learning experiments are small-scale, single-domain, and single-robot. This leads to a frequent tension in robotic learning: how can we learn generalizable robotic controllers without having to collect impractically large amounts of data for each separate experiment? In this paper, we propose RoboNet, an open database for sharing robotic experience, which provides an initial pool of 15 million video frames, from 7 different robot platforms, and study how it can be used to learn generalizable models for vision-based robotic manipulation. We combine the dataset with two different learning algorithms: visual foresight, which uses forward video prediction models, and supervised inverse models. Our experiments test the learned algorithms' ability to work across new objects, new tasks, new scenes, new camera viewpoints, new grippers, or even entirely new robots. In our final experiment, we find that by pre-training on RoboNet and fine-tuning on data from a held-out Franka or Kuka robot, we can exceed the performance of a robot-specific training approach that uses 4x-20x more data 引用
人にとっての当たり前が、ロボットには当たり前でない
今までスルーしていましたが、ロボットに服をたたむ作業は現状でもかなり難しい作業(技術)となっています。 アニメ等でみてる限りでは、ロボットは何でもできそうに思えるのですが、 この2022年をもってしてもロボットができる事はかなり少ないです。(開かれた空間において)
再度言いますが「ロボットは人間が想像するよりも、そして人間が当たり前にできる事」を未だにできません。
例えばどんなの?やロボット工学の現状はどうなの?と気になる方は、 「#ロボット工学の未解決問題」にアクセスしてみてください。
主にロボット系で著名な梶田先生等がツイートしておられます。
10年前のツイート。予想通りに想像を超えてAI・ロボティクス技術は激変した。
— Shuuji Kajita (@s_kajita) March 25, 2022
そして「実用的な知能ロボット」は依然、我々の目の前に険しい壁として立ちはだかっている。#ロボット工学の未解決問題 https://t.co/sbmekPTl1P
ツイートを見ていくうちに、ロボットってまだこんな事もできないの?となると思います。 (勘違いして欲しくないために一言添えますが、私はこれまでの研究や技術開発をリスペクトしています。 本当にロボットを動かすのは難しいです。でも、難しいからこそ、やりがいがあります)
遠隔操作によるロボットのデータ収集
では、難しいのはなんとなくわかったけど、 服をたたむ等のロボットデータ集めるのがそもそも無理なのでしょうか?
いえ、人力でJoyスティクがついたコントローラ等で遠隔操作等を行えばある程度集めることができます。
では想像してください。
- ゲームセンターのUFOキャッチャーとかって難しくないですか?
- 触れた事のないゲーム機で遊ぶ時、コントローラの操作に戸惑いがありませんか?
少しロボットと異なりますが、だいたい同じで、 ロボットの遠隔操作は玄人になればある程度「馴れ」で色々できるのですが、 「素人(ロボット操作未経験者)」にはかなり難しい(うまく服をたためない等)です。
余談ですが、こんな遠隔操作手法もあります。 引用:roboturk_1
あとは個人的に「バイラテラル制御」もかなり遠隔操作には良いと思います。(私も研究で活用しています。)
かなり長々記載してしまいましたが、 このようなロボティクス領域においてデータ収集の大変さは、RT-1の論文内でも以下のように記載してあります。
First, there’s the lack of large-scale and diverse robotic data, which limits a model’s ability to absorb a broad set of robotic experiences. Data collection is particularly expensive and challenging for robotics because dataset curation requires engineering-heavy autonomous operation, or demonstrations collected using human teleoperations.
まさに、そう!という感じです。
RT-1:ロボットデータ収集
かなり長い前置きになってしまい申し訳ございませんが、 RT-1の論文でのロボットデータ収集についてを記載します。 以下の様な環境でデータ収集しています。 EDRが沢山いて、とても広そうな実験環境ですね!
RT-1:ロボットデータ収集方法
では、どのようにデータ収集したのでしょうか?
RT-1の論文では以下の様な記載がありました。
We used demonstrations provided by humans through remote teleoperation, and annotated each episode with a textual description of the instruction that the robot just performed. The set of high-level skills represented in the dataset includes picking and placing items, opening and closing drawers, getting items in and out drawers, placing elongated items up-right, knocking objects over, pulling napkins and opening jars. The resulting dataset includes 130k+ episodes that cover 700+ tasks using many different objects.
Demonstrations are collected with direct line-of-sight between operator and robot using 2 virtual reality remotes. We map remote controls onto our policy action space to preserve consistency of the transition-dynamics. 3D position and rotational displacements of the remote are mapped to 6d displacements of the robot tool. The x, y position of the joystick is mapped to a turning angle and driving distance of the mobile base. We compute and track trajectories to the target poses that we obtain from the joystick commands.
要するに、2 virtual reality remotesでロボットを人が操作しているみたいです。(詳細は不明でした)
恐らくこの動画(BC-Z)のような事ではないかなと思っています。 人がVRコントローラでロボットを操作していますね。(動画には開始時間を設定しています)
RT-1:ロボットデータ収集数
上の方法でどのくらいまでデータを収集したのでしょうか?
RT-1の論文では以下の様な記載がありました。
We utilize a dataset that we gathered over the course of 17 months with a fleet of 13 robots, containing ∼130k episodes and over 700 tasks, and we ablate various aspects of this dataset in our evaluation.
The resulting dataset includes 130k+ episodes that cover 700+ tasks using many different objects.
- picking
- placing items
- opening and closing drawers
- getting items in and out drawers
- placing elongated items up-right
- knocking objects over
- pulling napkins
- opening jars
要するに、
- デモ数:130k
- 使用ロボット(EDR):13台
- Task数:744
- 収集期間:17ヶ月
- 多様なタスク
との事です。
この論文見た時に、データ量と17ヶ月という数字に驚きでした。 かなり凄いですね。
以下の図は横軸が期間で縦軸がタスクの成功率とタスクの数を表してるのですが、 17ヶ月の時を超えて、90%以上の成功率を叩き出していることが読み取れます。 タスク数も741と凄いですね。
- タスクイメージは以下の図で少しイメージがつくかもしれません。
RT-1:ロボットデータ収集数(応用編)
ここは冒頭でも述べましたが、
RT-1でおもしろかった所で、他のロボット(論文ではKUKA)、Sim2Real,Simデータを活用可能することでタスク成功率が向上したことです。
KUKAのデータはQT-Optが採用されています。
これのどこが凄いかと言いますと、 異構造のロボットであっても観測データを使用できるかもしれないという事です。 今回はEDRの大量のデータの中に、KUKAという異構造のロボットから取得したデータを追加しています。 前半で散々前置きが長かったのは、これを少し強調したかったからでした。(個人的にこの現象はテンションが上がりました。)
ですが、まだ実機ではEDRのアームとKUKA IIWAアームでしか検証できていないので、 他のロボット(自由度が異なる、行動可能範囲の違いなど)でも可能なのかが気になります。(書いてないだけかもしれませんが)
RT-1:モデル
色々と散々述べてきましたが、 RT-1のコアとなるモデルについてみていきます。
RT-1モデル概要
RT-1の入出力関係は
- input : images and natural language instructions
- output: action tokens
となっています。
モデルの流れとしては
- Step1: images and natural language instructionsを入力
- Step2: FiLM EfficientNet
- Step3: TokenLearner
- Step4: Transformer
- Step5: action (arm and base)を出力
となっています。
以下のようにTokenとして処理をしています。
- Instruction and image tokenization
- TokenLearner
- Transformer
- Action tokenization
Tokenはこれを見ればわかるはずです。
RT-1の実装も見てみましたが、Tokenの処理はここに記載されています。 論文もそうですが、実装をおうのも良いです。(疑問がなくなるかもです。)
オープンソースに感謝!!!(2022年12月14日現在では割とエラーがでてる人が多いみたいです。そのうち解決されそうですが。)
RT-1モデル詳細
RT-1のモデルの詳細は以下の図です。
Instruction and image tokenization(FiLM EfficientNet-B3)
ImagesにEDRが取得した6枚のRGB画像(解像度300×300)が入ります。 ImageNet pretrained EfficientNet-B3 modelを使用して、 9×9×512にmappingしています。
Instructionという所に、自然言語が入ります 自然言語処理はUniversal Sentence Encoder(USE)で処理されます。(Universal Sentence EncoderはSTSbenchmarkで高い精度を達成。異なる言語でも同じベクトル空間上にマップされるので言語の違いを意識する必要がないです) *APIもありますね。 USEで処理後にはidentity-initialized FiLM layers等を経由。
最終的に以下のようになります。
RT-1’s image and instruction tokenization via FiLM EfficientNet-B3 is a total of 16M parameters, with 26 layers of MBConv blocks and FiLM layers, which output 81 vision-language tokens.
TokenLearner
To further compress the number of tokens that RT-1 needs to attend over and thus speed up inference, RT-1 uses TokenLearner (Ryoo et al., 2021). TokenLearner is an elementwise attention module that learns to map a large number of tokens into a much smaller number of tokens. This allows us to soft-select image tokens based on their information, passing only the important token combinations to the subsequent Transformer layers. The inclusion of TokenLearner subsamples the 81 visual tokens that come out of the pre-trained FiLM-EfficientNet layers to just 8 final tokens that are then passed on to our Transformer layers.
ということなので、TokenLearnerを使用して、 8tokens×512までにしていますね。
Transformer
These 8 tokens per-image are then concatenated with the other images in the history, forming 48 total tokens (with added position encoding) to be fed into the Transformer backbone of RT-1. The Transformer is a decoder-only sequence model with 8 self-attention layers and 19M total parameters that outputs action tokens.
ということなので、Transformerで処理していきます。 ここからはほとんどTransformerのお話になるので、論文等をご覧ください!
☆Transformer参考資料
牛久さんの動画が個人的にわかりやすかったです。
- [DL輪読会]Attention Is All You Need
色々と実際に動かしてみると、楽しいです。(解説をサボってすみません。。。)
RT-1:実験
RT-1の論文で示されている実験についてみていきます。 詳細は論文をご覧ください! 要確認所をとりあげます。
Our experiments seek to answer the following questions:
- Can an RT-1 learn to perform a large number of instructions, as well as to generalize in zero shot to new tasks, objects and environments? >(Section 6.2)
- Can we push the resulting model even further by incorporating heterogeneous data sources, such as simulated data or data from different robots? (Section 6.3)
- How do various methods generalize to long-horizon robotic scenarios? (Section 6.4)
- How do generalization metrics change with varying amounts of data quantity and data diversity? (Section 6.5)
- What are the important and practical decisions in the design of the model and how do they affect performance and generalization? (Appendix Section D.4)
- Gato BC-Zとの違い
- Gato is, similarly to RT-1, based on a Transformer architecture, but varies from RT-1 in multiple aspects.
- First, it computes image tokens without the notion of language and each image token embedding is computed separately for each image patch, as opposed to early language fusion and global image embedding in our model.
- Second, it does not use a pre-trained text embedding to encode the language string. It also does not include inference time considerations that are necessary for real robots as discussed in Sec. 5.1 such as TokenLearner and the removal of auto regressive actions.
改良点
- In order to run Gato on real robots at a high enough frequency, we also limit the size of the model compared to the original publication, which was 1.2B parameters (resulting in on robot inference time of 1.9s), to be of similar size to RT-1 (37M parameters for Gato vs. 35M for RT-1). BC-Z is based on a ResNet architecture, and was used in SayCan (Ahn et al., 2022).
We conduct many ablations of the model (common) and the data (less common), where we have some interesting findings. For example, we find that data diversity >> data quantity and that modeling actions auto-regressively doesn’t help much but makes the model considerably slower. pic.twitter.com/Qg3LZB3g6X
— Karol Hausman (@hausman_k) December 13, 2022
RT-1:限界や課題や対策
RT-1の限界
RT-1の課題等は以下のように論文では述べられています。
While RT-1 presents a promising step towards large-scale robot learning with an data-absorbent model, it comes with a number of limitations.
- First, it is an imitation learning method, which inherits the challenges of that class of approaches such as the fact that it may not be able to surpass the performance of the demonstrators.
- Second, the generalization to new instructions is limited to the combinations of previously seen concepts and RT-1 is not yet able to generalize to a completely new motion that has not been seen before.
- Lastly, our method is presented on a large but not very dexterous set of manipulation tasks. We plan to continue extending the set of instructions that RT-1 enables and generalizes to to address this challenge.
つまり、
- 模倣学習である→性能の限界がある(かもしれない)
- 見たことのない全く新しい動作に汎化することはできない(かもしれない)
- 器用な作業ができない(学習データに含めていない)
との事です。
RT-1の今後
As we explore future directions for this work, we hope to scale the number of robot skills faster by developing methods that allow non-experts to train the robot via directed data collection and model prompting. While the current version of RT-1 is fairly robust especially to distractor objects, its robustness to backgrounds and environments could be further improved by greatly increasing the environment diversity. We also hope to improve the reaction speeds and context retention of RT-1 through scalable attention and memory.
今後の道筋も少し示してくれていますね。
改めて….
こちらの動画のイメージを少しでも読む前より、理解して頂けれていたら、 とても嬉しいです。
To address these challenges, we propose the Robotics Transformer 1 (RT-1), a multi-task model that tokenizes robot inputs and outputs actions (e.g., camera images, task instructions, and motor commands) to enable efficient inference at runtime, which makes real-time control feasible. This model is trained on a large-scale, real-world robotics dataset of 130k episodes that cover 700+ tasks, collected using a fleet of 13 robots from Everyday Robots (EDR) over 17 months. We demonstrate that RT-1 can exhibit significantly improved zero-shot generalization to new tasks, environments and objects compared to prior techniques. Moreover, we carefully evaluate and ablate many of the design choices in the model and training set, analyzing the effects of tokenization, action representation, and dataset composition. Finally, we’re open-sourcing the RT-1 code, and hope it will provide a valuable resource for future research on scaling up robot learning.
おわりに
以上、RT-1はなんでもできそうに見えてしまいますが、 個人的にはまだまだできない事が多い様に感じます。
しかしながら、ロボットが人の自然言語指示で動作する未来が少し垣間見えたような気がして、 ワクワクしました! 何か物をとってきてくらいなら、できてしまいそうな気がします。
今後は器用な動作やAction Spaceの改善にも期待したいと個人的に思っています。
改めて言いますが、 「ロボットは本当に人間が想像するよりも、 そして人間が当たり前にできる事をできない」です。
これらが解決できる日々へ向けて、私も更に研究や開発に励んでいこうと思います。
長々した記事となってしまいましたが、最後までお読み頂きありがとうございました。
少しでも楽しんで頂ければとても嬉しいです! (まだまだ学びが足りないので精進していきます!)
TRAIL:ロボカップ世界大会へ向けた挑戦
最後に、、、
東京大学松尾豊研究室のサブグループであるTRAIL からのお知らせです。
来年のロボカップ世界大会に向けた技術紹介動画(Qualification Video)を公開しました!
モバイルマニピュレータHSRを使って,家庭環境下での生活支援タスクを実行している模様をご覧いただけます.
学部1年生がHSR動かして、なおかつ動画作成までしました!
そして、「基盤モデル」ももちろんロボット活用しております。
ぜひご覧ください!
来年のロボカップ世界大会に向けた技術紹介動画(Qualification Video)を公開しました!
— TRAIL (Tokyo Robotics and AI Lab) (@trail_ut) December 13, 2022
モバイルマニピュレータHSRを使って,家庭環境下での生活支援タスクを実行している模様をご覧いただけます.https://t.co/vv6lgFe3UO
☆Youtube
☆Advent Calendar 2022 「基盤モデル×Robotics」
「基盤モデル×Robotics」も引き続きよろしくお願いします!
【募集📅:Advent Calendar2022(基盤モデル×Robotics🤖)】
— 東京大学 松尾研究室 (@Matsuo_Lab) November 11, 2022
・投稿募集🎉
東京大学松尾研究室にて、Advent Calendar2022を『基盤モデル×Robotics🤖』で開催します😊
※松尾研外からのご参加をお待ちしております!🎄
・投稿先📅
↓詳細や投稿先はこちらへ⛄https://t.co/yPorYPLyAE
最後までお読み頂きありがとうございました。
12月15日は私の誕生日…もしやRT-1はGoogleからの誕生日プレゼント?(失礼しました) ↩︎